Recent studies and articles have questioned the ability of AI tools to fairly rank job applicants, suggesting potential biases in their evaluations. A recent piece from HR Dive points out some concerns regarding bias in AI-driven applicant ranking systems. However, while these findings highlight important cautionary points, they don’t capture the full picture. When designed and used correctly, AI can actually improve fairness and reduce human biases in hiring.
Here, we’ll explore why AI has the potential to offer unbiased applicant rankings, examine the factors that contribute to AI accuracy, and look at statistics supporting AI’s role in fair hiring practices.
Understanding the Flaws in Current AI Bias Studies
- Limited Data Sets in Studies: Many studies assessing AI biases use small or unrepresentative data sets, leading to conclusions that may not reflect real-world applications. For example, an AI model trained on a narrow dataset could indeed show bias, but robust AI tools rely on large, diverse datasets that mitigate this risk.
- Lack of Context on Model Training: AI models used in hiring rely on training data. If studies do not account for variations in training datasets, it’s easy to overlook how models can be built to be fair. The lack of transparency in some reports doesn’t always clarify the parameters or the diversity of data sources used in AI model training.
- Human Bias and Mislabeling in Training Data: Some AI bias findings overlook how human input in training data affects outcomes. If historical data was influenced by human bias, the AI could mirror this. However, modern AI systems use techniques to address and minimize these biases, a factor often omitted in studies.
Evidence Supporting AI’s Potential to Reduce Bias
Despite criticisms, AI has shown a significant ability to provide fair and unbiased hiring outcomes when applied responsibly. Here’s why:
- AI Can Reduce Human Bias in Screening
Research shows that humans are subject to both conscious and unconscious biases in hiring. A study by Harvard Business Review found that 58% of hiring managers admit to making snap judgments based on a candidate’s appearance or background. AI, on the other hand, evaluates candidates solely on pre-defined, objective criteria, removing the influence of personal biases. - Data-Driven Fairness
According to a report by the National Bureau of Economic Research, AI-assisted hiring resulted in a 30% increase in job placements for underrepresented candidates in tech roles compared to human-only screening processes. This is because AI is programmed to prioritize skills, qualifications, and experience rather than subjective qualities that might lead to bias. - Greater Diversity Through Skill-Based Evaluations
LinkedIn’s 2022 Workplace Learning Report found that companies using AI to screen applicants saw a 47% increase in diversity metrics, as AI-based systems focus on skill sets rather than cultural or personal similarities that might influence human decisions. By evaluating candidates on capabilities alone, AI can lead to a more diverse and inclusive workforce.
Steps to Ensure AI Fairness in Hiring
For AI tools to reach their unbiased potential, organizations need to follow best practices in AI design and implementation. Here are some strategies to maximize fairness:
- Using Diverse Training Data
High-quality AI systems rely on diverse, representative data to train models. When companies ensure that their training data reflects a broad range of demographics, backgrounds, and experiences, AI tools can avoid bias more effectively. - Regular Bias Audits
Companies using AI hiring tools should conduct regular audits to identify and address potential biases in AI outputs. According to McKinsey, companies that perform routine AI audits report a 35% higher likelihood of achieving fair hiring outcomes than those that do not. - Transparency in AI Algorithms
Transparent AI algorithms, where users understand what criteria the AI evaluates, help ensure objectivity. A study by MIT Sloan found that transparency in AI systems increased the trust and reliability of AI-driven decisions by 42%, as it enables organizations to monitor and correct for biases effectively.
Why AI Can Be the Solution, Not the Problem
AI hiring tools, when carefully implemented, offer a scalable way to evaluate candidates fairly and efficiently. Unlike humans, who may be influenced by personal biases or preferences, AI focuses on measurable qualities, like skills and experience, allowing companies to rank applicants based solely on relevant qualifications.
Critics often focus on instances where AI has demonstrated bias, but these cases typically arise from flawed data or poorly designed algorithms, not the AI itself. With transparent practices, diverse training data, and regular monitoring, AI can indeed improve fairness in hiring.
Key Takeaways
- AI systems can eliminate many of the human biases that lead to unfair hiring practices by focusing on objective criteria.
- Studies showing AI bias often lack robust data or do not account for advanced bias-mitigation strategies.
- Evidence shows that companies using AI tools see increases in diversity and reduced bias, as AI emphasizes skills and experience over subjective factors.
- Properly designed and monitored AI systems can significantly enhance fair hiring practices, driving better outcomes for both companies and candidates.
Conclusion
While concerns about AI bias in hiring are valid and should be addressed, they don’t tell the whole story. AI has the potential to revolutionize hiring, offering fairer and more objective applicant rankings than traditional methods. With CloudApper’s AI platform, companies can leverage unbiased, skill-based evaluations to identify top candidates while minimizing human biases, creating a fairer and more inclusive hiring process.
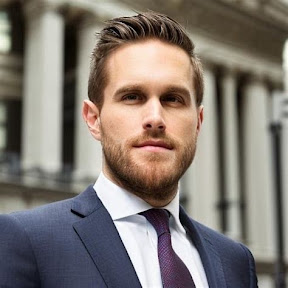
Darren Trumbler is a versatile content writer specializing in B2B technology, marketing strategies, and wellness. With a knack for breaking down complex topics into engaging, easy-to-understand narratives, Darren helps businesses communicate effectively with their audiences.
Over the years, Darren has crafted high-impact content for diverse industries, from tech startups to established enterprises, focusing on thought leadership articles, blog posts, and marketing collateral that drive results. Beyond his professional expertise, he is passionate about wellness and enjoys writing about strategies for achieving balance in work and life.
When he’s not creating compelling content, Darren can be found exploring the latest tech innovations, reading up on marketing trends, or advocating for a healthier lifestyle.